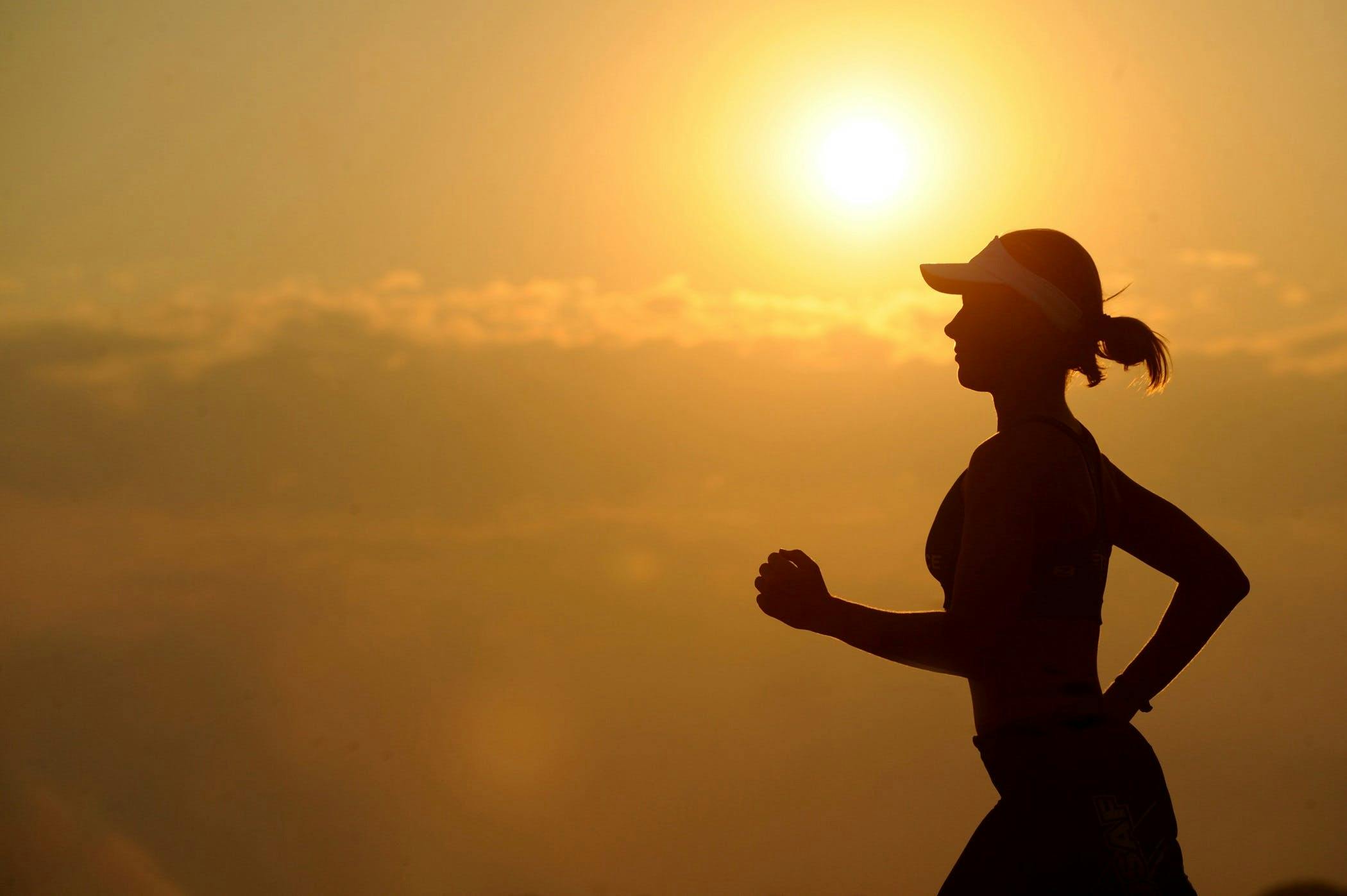
Does the Quantified-Self Lead to Behavior Change?
In 2006, marketing commentator, Michael Palmer said “Data is just like crude. It’s valuable, but if unrefined it cannot really be used” [15]. Over a decade on, our lives are more saturated with data than ever, but we still seem far from harnessing its full potential.
Health and well-being is one area in particular where this issue is very prevalent. In recent years, fitness technologies have produced a plethora of data for individuals who want to change, abandon, or adopt particular habits related to their health. Just like crude oil, health data has subsequently become hugely abundant.
However, if we don’t learn how to best navigate and refine the large amounts of data offered by this technology, then it will be difficult to help people use it for the betterment of their health and well-being. This article explores how these difficulties can be overcome, and highlights how behavioral science can untangle the complex relationship between technology and long-lasting behavior change.
References
[1] Bargh, J. A. (1994). The four horsemen of automaticity: Awareness, intention, efficiency, and control in social cognition. In R. S. Wyer & T. K. Srull (Eds.), Handbook of Social Cognition: Vol. 1 Basic Processes (pp. 1-40). Hove: Lawrence Erlbaum Associates.
[2] Bildl, S. (2014). Gamification of the quantified self. In Stokinger, T., Lindemann, P., Koelle, M., & Kranz, M. Fun, Secure, Embedded. Advances in Embedded Interactive Systems Technical Report – Summer 2014, 2(3), 5-10.
[3] Bennet, B., & Murdock, J. R. (1962). The serial position effect of free recall. Journal of Experimental Psychology, 64(5), 482-488.
[4] Calvo, R. A., & Peters, D. (2013). The irony and re-interpretation of our quantified self. Proceedings of the 25th Australian Computer-Human Interaction Conference on Augmentation, Application, Innovation, Collaboration – OzCHI 13.
[5] Deterding, S. 2012. Gamification: Designing for motivation. Interactions, 19(4), 14-17.
[6] Erskine, J. A., Georgiou, G. J., & Kvavilashvili, L. (2010). I suppress, therefore I smoke: effects of thought suppression on smoking behavior. Psychological science, 21(9), 1225–30.
[7] Fritz, T., Huang, E. M., Murphy, G. C., Zimmerman, T. (2013) Persuasive technology in the real world: A study of long-term use of activity sensing devices for fitness. Proceedings of the ACM Conference on Human Factors in Computing Systems – CHI 14.
[8] Harrison, D., Marshall, P., Bianchi-Berthouze, N., & Bird, J. (2015). Activity tracking. Proceedings of the 2015 ACM International Joint Conference on Pervasive and Ubiquitous Computing – UbiComp 15.
[9] Hayward, J., Chansin, G., & Zervos, H. (2017) Wearable technology 2017-2027: Markets, Players, Forecasts. Retrieved September 27th 2017, from https://www.idtechex.com/research/reports/wearable-technology-2017-2027-markets-players-forecasts-000536.asp.
[10] Klasnja, P., Consolvo, S., Mcdonald, D. W., Landay, J. A., & Pratt, W. (2009). Using mobile and personal sensing technologies to support health behavior change in everyday life: Lessons learned. AMIA Annual Symposium Proceedings, 2009, 338-342.
[11] Lupton, D. (2014). Self-Tracking Modes: Reflexive Self-Monitoring and Data Practices. Retrieved September 27, 2017, from https://papers.ssrn.com/sol3/papers.cfm?abstract_id=2483549
[12] Lupton, D. (2016). The diverse domains of quantified selves: self-tracking modes and dataveillance. Economy and Society, 45(1), 101-122.
[13] Niedenthal, P. M. (2007). Embodying emotion. Science, 316(5827), 1002–1005.
[14] Norman, D. A. (2009). THE WAY I SEE IT. Memory is more important than actuality. Interactions, 16 (2), 24-26.
[15] Palmer, M. (2006). Data is the new oil. 2006. Retrieved September 27th, 2017, from: https://ana.blogs.com/maestros/2006/11/data_is_the_new.html.
[16] Patel, M. S., Asch, D. A., & Volpp, K. G. (2015). Wearable Devices as Facilitators, Not Drivers, of Health Behavior Change. Jama, 313 (5), 459-460.
[17] Pinder, C., Vermeulen, J., Beale, R., & Hendley, R. (2015). Exploring Nonconscious Behavior Change Interventions on Mobile Devices. Proceedings of the 17th International Conference on Human-Computer Interaction with Mobile Devices and Services Adjunct – MobileHCI 15.
[18] Renfree, I., Harrison, D., Marshall, P., Stawarz, K., & Cox, A. (2016). Don’t Kick the Habit. Proceedings of the 2016 CHI Conference Extended Abstracts on Human Factors in Computing Systems – CHI EA 16.
[19] Sjöklint, M., Constantiou, I. D., & Trier, M. (2015). The Complexities of Self-Tracking – An Inquiry into User Reactions and Goal Attainment. SSRN Electronic Journal.
[20] Stawarz, K., Cox, A. L., & Blandford, A. (2015). Beyond Self-Tracking and Reminders. Proceedings of the 33rd Annual ACM Conference on Human Factors in Computing Systems – CHI 15.
[21] Swan, M. (2015). Connected Car: Quantified Self becomes Quantified Car. Journal of Sensor and Actuator Networks, 4 (1), 2-29.
[22] Wood, W., & Neal, D. (2009). The habitual consumer. Journal of Consumer Psychology, 19, 579-592.
[23] Yang, R., Shin, E., Newman, M. W., & Ackerman, M. S. (2015). When fitness trackers don’t fit. Proceedings of the 2015 ACM International Joint Conference on Pervasive and Ubiquitous Computing – UbiComp 15.
About the Author
Zoe Adams
Zoe is a PhD candidate in Linguistics at Queen Mary University of London. She is bridging the gap between public health and language attitudes by studying how British accents affect the persuasiveness of public health interventions. Her interests include consumer psychology, attitude change, and stereotyping.
About us
We are the leading applied research & innovation consultancy
Our insights are leveraged by the most ambitious organizations
















“
I was blown away with their application and translation of behavioral science into practice. They took a very complex ecosystem and created a series of interventions using an innovative mix of the latest research and creative client co-creation. I was so impressed at the final product they created, which was hugely comprehensive despite the large scope of the client being of the world's most far-reaching and best known consumer brands. I'm excited to see what we can create together in the future.
Heather McKee
BEHAVIORAL SCIENTIST
GLOBAL COFFEEHOUSE CHAIN PROJECT
OUR CLIENT SUCCESS
$0M
Annual Revenue Increase
By launching a behavioral science practice at the core of the organization, we helped one of the largest insurers in North America realize $30M increase in annual revenue.
0%
Increase in Monthly Users
By redesigning North America's first national digital platform for mental health, we achieved a 52% lift in monthly users and an 83% improvement on clinical assessment.
0%
Reduction In Design Time
By designing a new process and getting buy-in from the C-Suite team, we helped one of the largest smartphone manufacturers in the world reduce software design time by 75%.
0%
Reduction in Client Drop-Off
By implementing targeted nudges based on proactive interventions, we reduced drop-off rates for 450,000 clients belonging to USA's oldest debt consolidation organizations by 46%